- Sep 15, 2009 The Bike Smart Program. The Bike Smart program is an eHealth product which utilizes video, animations, and still images to train children in kindergarten to third grade the key skills for bicycle safety.
- Highway Safety Program Guideline No. 14 Pedestrian and Bicycle Safety (2006). Recognizing the importance of emergency medical services (EMS) as a critical component to addressing traffic safety, the Team added a section focused on EMS. The Team conducted the Michigan Pedestrian and Bicycle Safety Program Assessment at the.
Bike LA Safety Training is the type of program that takes into consideration the needs of the urban lower social-economic class. Bicycle safety programs for the very young (ages 0 to 5) are not as representative. In 2001, children 15 years and younger accounted for 59 percent of all bicycle-related injuries seen in emergency departments across the U.S. Bike safety tips Make sure your child wears a helmet at all times when cycling, skating or skateboarding. NHTSA’s bicycle safety programs focus on the behaviors of bicyclists and motorists to reduce injuries and fatalities on our nation’s roadways. NHTSA is dedicated to promoting safe motorist and bicyclist behavior through education and enforcement efforts. The Bike Smart Program. The Bike Smart program is an eHealth product which utilizes video, animations, and still images to train children in kindergarten to third grade the key skills for bicycle safety.
Abstract
Objective The purpose of this study was to evaluate the “Bike Smart” program, an eHealth software program that teaches bicycle safety behaviors to young children. Methods Participants were 206 elementary students in grades kindergarten to 3. A random control design was employed to evaluate the program, with students assigned to either the treatment condition (Bike Smart) or the control condition (a video on childhood safety). Outcome measures included computer-based knowledge items (safety rules, helmet placement, hazard discrimination) and a behavioral measure of helmet placement. Results Results demonstrated that regardless of gender, cohort, and grade the participants in the treatment group showed greater gains than control participants in both the computer-presented knowledge items (p > .01) and the observational helmet measure (p > .05). Conclusions Findings suggest that the Bike Smart program can be a low cost, effective component of safety training packages that include both skills-based and experiential training.
C A R E S Of Farmington Hills
Introduction
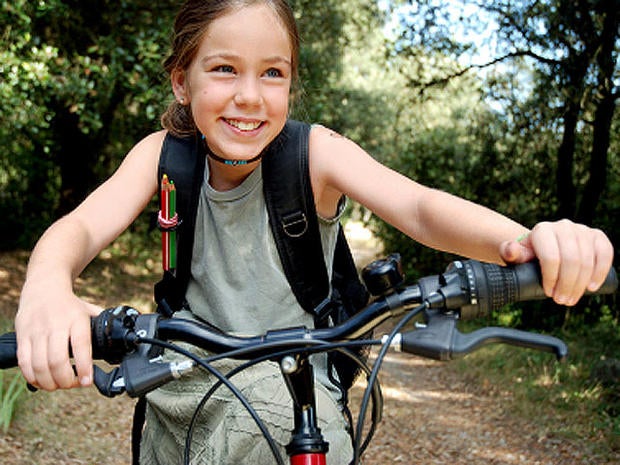
Traffic-related injuries are a leading cause of death and disability for children in America (CDC, 2005a) and many are bicycle-related. One-fifth of all bicycle-related injuries occur in children 15 years and younger (National Highway Traffic Safety Administration, 2006), with about 85% involving collisions with motor vehicles (CDC, 2005b). In addition to fatalities, bicycle crashes are a common cause of serious brain injury in children. Approximately one-half of children under age 15 hospitalized after a bicycle crash are diagnosed with traumatic brain injury (National SAFE KIDS Campaign, 2004). Reducing bicycle injuries in children requires a multifaceted approach to change child behaviors by increasing safe riding behavior, helmet adherence, and avoidance of hazardous situations (Finnoff, Laskowski, Altman, & Diehl, ; Gielen & Sleet, ; Rivara, Thompson, & Thompson, ).
Approaches to improve children's safety knowledge and behavior are ideally addressed from multiple avenues, including environmental changes, legislation, parent counseling, and educational programs (Chen, Kresnow, Thomas, & Dellinger, ; Haileyesus, Annest, & Dellinger, ; Royal, Kendrick, & Coleman, ). Most efforts have focused on increased bike helmet use, which significantly decreases the incidence of bicycle-related head injuries (Finvers, Strother, & Mohtadi, ; Rivara et al., ; Thompson, Rivara, & Thompson, ). However, despite public health measures, legislation, and children's knowledge of the injury reducing effects of helmet usage, observational studies reveal that between one-half and three-quarters of children under age 14 continue to ride unprotected (Finnoff et al., ; Van Houten, Van Houten, & Malenfant, ). For children who do wear helmets, poor fit or placement can lead to injuries to the forehead and face and result in brain injury (Ching et al., ; Rivara, Astley, Clarren, Thompson, & Thompson, ).
In addition to encouraging bicycle helmet use, safety education is an important component of injury reduction strategies. Addressing the risks associated with traveling in traffic is particularly relevant, as both physical environments and children's behavior increase the risk of injury (Barton, Schwebel, & Morrongiello, ). Intersections pose substantial risk for pedestrians and cyclists due to the presence of cars turning in multiple configurations (Wang & Nihan, ), and children aged 5–9 years are frequently injured in intersections due to unsafe crossing behaviors (Morrongiello, 2003). This can be addressed through instruction in interpreting complex traffic patterns and riding environments (Peterson & Schick, ), as well as basic safety rules (e.g., riding with hands on the handlebars).
Most injury prevention educational programs are classroom-based, and use book, video, and lecture formats to teach safety rules and general safety information to children (Kirsch & Pullen, ; Nagel, Hankenhof, Kimmel, & Saxe, ). While generally low cost to administer, most of these instructional methods fail to result in measurable behavioral outcomes (e.g., Luria, Smith, & Chapman, ). Several large scale community wide campaigns have been effective in reducing pedestrian and bicycle-related injury rates (e.g., Hotz et al., ; Morris, Trimble, & Fendley, ; Rivara et al., ). These efforts have been multi-faceted, including helmet give-away and bicycle safety education, making it difficult to determine the proportion of effects attributed to a safety education component. Recently, safety education programs utilizing active learning and feedback in a brief intervention have demonstrated promise. For example, Morrongiello and Kiriakou () showed that a one session pedestrian safety program involving role playing, problem solving, and inference exercises led to significant changes in students’ safety knowledge and increase in self-reported safety behaviors.
A cost-effective approach to active bicycle safety instruction is the use of eHealth programs. Use of the internet to deliver health education to youth has been used effectively in the areas of smoking cessation (Buller et al. ; Walters, Wright & Shegog, ), self-management of health conditions (Stinson, Wilson, Gill, Yamad & Holt, 2008) and other pediatric health-related behaviors (Hornung et al., ; Krishna et al., ). In the context of childhood injury prevention, most work in the area of eHealth has been conducted using computerized (Glang, Noell, Ary, & Swartz, ) or virtual environments (Schwebel, Gaines, & Severson, ) to teach pedestrian safety. Computer technology allows: use of animations that remove irrelevant stimuli; gradual replacement of animations with semi-abstracted examples (e.g., actual photographs of intersections with animated cars) to increase generalization; and presentation of real-life examples of biking scenarios through video, thereby simulating natural environments. Furthermore, the application of empirically validated instructional design principles can ensure that those instructional programs which take full advantage of computer-based video training methods can effectively and efficiently teach these skills, and teach those skills in ways which will promote their generalization and maintenance (Engelmann & Carnine, 1982; Horner, McDonnell, & Bellamy, 1986).
The purpose of this study was to evaluate the “Bike Smart” program (Bike Smart, 2005), an eHealth software program aimed at training children in grades kindergarten to three key bicycle safety behaviors. This program was designed utilizing instructional design principals in order to improve upon traditional classroom instruction, and deliver bike safety training in an efficient and instructionally effective manner. Specifically, we hypothesized that participants who viewed the program would demonstrate increased ability to: discriminate safe and unsafe riding behaviors; identify hazards in the riding environment; judge the appropriate placement of a bicycle helmet; and demonstrate correct helmet placement on their own heads, when compared to participants in the control condition.
Methods
Participants
The study was conducted in a suburban school district over two, 1-week periods in the Pacific Northwest. The participants were 206 elementary students in kindergarten (n = 21), first grade (n = 62), second grade (n = 73), and third grade (n = 50) with 57% of the sample male and 42% female (1% of the sample did not report gender). Participants were recruited from two schools with 61% coming from School A and 39% from School B. Minority status and socioeconomic status (SES) was not collected at the participant level during this study, but rates were available at the school level. Minority status at Schools A and B was 40 and 34%, respectively. Both schools reported 77% of enrolled students qualified for free or reduced lunch, a proxy measure for SES.
The study was approved through the school district research approval process. Ten teachers (representing grades kindergarten to 3) in two elementary schools volunteered to have their classes participate in the study. A total of 246 students were identified as possible participants. Following the Human Subjects guidelines of the school district, several weeks prior to beginning the study, the teachers sent home information about the Bike Smart program and a passive consent form to parents of all students in their classes. The parents of two children declined participation and one child was absent on the day consent forms were sent home, leading to a participant pool of 243 students. In addition to parent consent, all student participants were informed verbally of the study purposes and procedures, and indicated their willingness to complete the project. Because the study's intervention was deemed to be normal education practices in a school setting by the Institutional Review Board, we did not obtain child assent.

The Bike Smart Program
The Bike Smart program is an eHealth product which utilizes video, animations, and still images to train children in kindergarten to third grade the key skills for bicycle safety. In videos, two actors in the target age and two young teens present instruction and positive messages about safety behavior, as children begin to use peers as referents for behavior at a young age (Morrongiello & Schwebel, 2008). The program consists of two learning units which include sections covering equipment safety, bike helmets, and riding skills (e.g., riding with only one rider on the bike). Following presentation of the concepts in each unit, the interactive program allows the student to analyze situations and receive feedback on their choices. For example, after viewing a segment on helmet placement, the student views positive and negative examples of helmet placement and determines if the helmet is placed appropriately. Each training example was designed to present the safety rules with description of the hazard posed by a particular behavior or threat. For example, in the section on crossing intersections, the student is shown a diagram of the intersection, placement of a threat vehicle, and animation of how the car can move in the rider's path. Knowledge test items in the program require the student to evaluate situations and answer yes or no to global (e.g., is this rider safe?) and specific (e.g., can this car turn in her path?) situations.
Program development
Content for the Bike Smart program was selected through a project advisory group consisting of parents, educators, state health and injury prevention specialists, school technology specialists, and traffic and safety officials. Key content areas identified through this process as most important were information on how to wear a helmet correctly and navigate in real-life environments. The program was developed using Macromedia Director, which uses a timeline-based approach to allow both user input and predetermined programming to control the content and feedback the user will receive at various points in the program. Once developed, we pilot tested the program with 78 children in the target age group to determine program revisions. Finally, accompanying parent/teacher materials were added to the program disc in pdf format (available from the authors). These materials included additional learning activities and outline of key instructional components.
Program Evaluation
A random control design was employed to evaluate the program. Teachers provided class lists of the 243 students who would participate and each student was assigned a participant number. The project coordinator randomly assigned students to either the treatment condition (Bike Smart) or the control condition (a video on childhood safety) using a computerized random number generator to assign students to each condition. We employed stratified randomization by grade of participant to balance the number of treatment and control participants in each grade. A sheet with each participant number was created for use during the evaluation to check each participant through the steps of the evaluation (e.g., computerized program, behavioral observation) based on their assigned condition.
The evaluation took place over 2 days. On the first day, research assistants checked a list of student names and participant numbers to access students’ evaluation sheets with their assigned conditions. Students were escorted to a computer laboratory in which they sat at circular tables of six students. The research assistants assigned computers (laptop computers with headphones to minimize distraction) to each student and started the pre-test for each student. Research assistants were not aware of each child's name or assigned condition prior to the day of evaluation, and students were not aware of their assignment prior to starting the program. Following the pre-test, students in the treatment condition completed Unit 1 of the Bike Smart program, and students in the control condition viewed the first half of a safety video (“Be Cool, Play it Safe;” Mish & Barker, 2000). Students in both groups spent approximately 40 min completing the pre-test assessment and the Bike Smart Unit 1 or computer-delivered safety video.
On the second day, students in both conditions spent approximately 20 min viewing either the treatment (Bike Smart Unit 2) or control video in the computer lab, then immediately completed the post-test. In order to ensure that any pre- to post-test differences on the computerized measures were not due to enhanced mouse skill gained in using the program (i.e., knowing how to locate and depress the mouse; selecting from a field of choices), students were pre-trained in using the mouse to navigate and select responses through a computerized mouse training segment. The pre-test followed directly after the mouse practice segment. No reported adverse events or side effects were experienced by the participants during the evaluation. Children took home copies of the parent materials after completing the study.
Outcome Measures
One observational and three computerized measures were used to evaluate the effectiveness of the Bike Smart program. For the computerized measures, the computer recorded each response and stored the data on a folder linked to each participant number. These data were objective and not subject to influence of knowing a participant's assigned condition.
Bike helmet score
A three-item observational measure assessed a student's skill at correctly putting on a bike helmet. Each student was asked to put on a bike helmet as if they were preparing to go for a bike ride. An observer scored them on whether or not the helmet was: placed correctly over the forehead (i.e., approximately two fingers width from eyebrows; was sitting straight across from ear to ear (i.e., the helmet was on straight); and the buckle was strapped. A total correct sum score (range of 0–3) was calculated at pre- and post-test. Children were not assessed for their ability to correctly fit the helmet by adjusting the buckles, as pilot testing and expert input indicated that children in the target age were physically unable to reliably manipulate the buckles and straps.
Helmet skill discrimination
A two-item computerized measure assessed a student's ability to correctly identify whether or not the person viewed on the computer screen was wearing their bike helmet correctly (e.g., too high on the forehead). Figure 1 shows an example of a helmet item. A total correct sum score (range of zero to two) was calculated at pre- and post-test.
Helmet discrimination item.
Safety rules
A four-item computerized measure assessed a student's ability to correctly identify whether or not the situation viewed in the program was safe. The first situation showed a proper hand signal a biker would use when stopping. The other three situations showed bikers who were: riding safely with a helmet, wearing no helmet, and riding while holding items in their hands. An example item is found in Figure 2. A total correct sum score (range of zero to four) was calculated at pre- and post-test.
Safety rules item.
Hazard discrimination
A 17-item computerized measure assessed a student's ability to identify hazardous situations viewed in the program. Items assessed dangerous cars at an intersection (e.g., a car turning left could cross the rider's path); hazards when riding on the side-walk (e.g., cars exiting a driveway); and hazards encountered when riding on the street (e.g., a car door opening) in a first person view. Figure 3 shows an example of a hazard item on the street. A total correct sum score (range of 0–17) was calculated at pre- and post-test.
Hazard discrimination item.
Inter-observer reliability
All observational helmet measures were coded by one trained observer, and reliability was established with an additional coder for 23% of the observations. Cohen's kappa coefficient (Cohen, 1960) was calculated at .62, indicating acceptable inter-observer agreement (Landis & Koch, ). Both observers were blind to the participants’ assigned conditions.
Sample size
Based on our prior studies (Glang, 1999) with the same outcome measures we expected large effect sizes for the helmet discrimination items and small to medium effects for hazard discrimination items. The small effect sizes in previous studies were determined not to be practically significant. Therefore we powered the current study to detect medium effect sizes. Based on power of .80 to detect a significant difference (p = .05, two-tailed) in proportions, 102 children were required for each condition.
Flow of participants
Figure 4 contains the flow of participants through the evaluation. Following assignment to each condition, a total of 15 treatment participants and 22 control participants’ data were excluded from analysis. Specifically, for 24 participants (13 treatment, 11 control) the data file stored on the computer was corrupted due to program malfunction. These data were excluded as we did not have a full set of computerized and observational data for those participants. In addition, 13 participants’ data were excluded from the analysis when it was determined that the participant had limited English proficiency (n = 2) or had cognitive impairments which affected learning (n = 11). Those students were not, however, excluded from participating with their classmates during the evaluation.
Participant flowchart.
Results
Preliminary Analysis
Prior to the main analysis all measures were screened for out-of-range values and distributional properties were examined. We verified that students in the two conditions did not differ significantly in terms of gender [χ2 (1, 206) = 0.04; p = .842], current grade [χ2 (3, 209) = 0.87; p = .828], or school attended [χ2 (1, 209) = 0.80; p = .372], which suggests that randomization created initially equivalent groups. No attrition occurred from the pre- to the post-test assessments
Intervention Main Effects
A doubly multivariate repeated measures analysis of variance (MANOVA) was used to determine if there was an overall significant pre-test to post-test difference between study conditions on the observational and three computerized measures. Condition was a two-level between-subjects factor and time was a two-level within-subjects factor. The time × condition interaction tests whether participants in one condition show significantly greater increases on the outcomes than participants in the other condition at the post-test follow-up assessment. Therefore, the time × condition interaction represents a direct test of the effects of the intervention condition relative to the control condition. Based on Pillai's test a significant overall differential time × condition by time effect [F(4, 201) = 91.29; p < .001] with a large multivariate effect size (η2 = .65) was found. The significant overall effect warranted separate pre- to post-test investigation of each scale to help determine which measure(s) significantly contributed to the overall differences between study conditions.
Next, a repeated measures ANOVA model that contrasted the treatment and control conditions at post-test was run for each of the four outcome measures. Like the omnibus test described above condition was a two-level between-subjects factor and time was a two-level within-subjects factor. The direct test of the intervention, the time × condition interaction, was significant for each measure and the treatment condition showed significantly more improvement from pre-test to at post-test compared with the control participants (see Table I). The intervention effects for the three computerized measures were associated with medium to large effect sizes, but the observational measure was associated with a small effect size. Next, higher order three-way interactions involving grade and sex were examined to determine whether or not these demographic characteristics moderated the significant time × condition effects, but no significant interactions were found.
A R E S Ham Radio
Table I.
Descriptive Statistics, Significance Levels, and Effects Sizes for the Direct Test of Intervention Effects
Pre-test | Post-test | ||||||||||||||
---|---|---|---|---|---|---|---|---|---|---|---|---|---|---|---|
Treatment (n = 107) | Control (n = 99) | Treatment (n = 107) | Control (n = 99) | Condition × Time | |||||||||||
Outcome measure | M | SD | R | M | SD | R | M | SD | R | M | SD | R | F | p | η2 |
Observational bike helmet score | 2.2 | 0.8 | 0–3 | 2.1 | 0.7 | 0–3 | 2.4 | 0.7 | 1–3 | 2.0 | 0.7 | 0–3 | 7.28 | .008 | .03 |
Computerized measures | |||||||||||||||
Helmet skill discrimination | 0.5 | 0.6 | 0–2 | 0.5 | 0.6 | 0–2 | 1.8 | 0.4 | 0–2 | 0.5 | 0.6 | 0–2 | 230.31 | <.001 | .53 |
Safety rules | 2.7 | 0.7 | 0–4 | 2.7 | 0.7 | 0–4 | 3.7 | 0.7 | 1–4 | 2.7 | 0.7 | 0–4 | 67.46 | <.001 | .25 |
Hazard discrimination | 9.9 | 2.3 | 3–13 | 10.0 | 2.4 | 4–14 | 14.6 | 1.2 | 6–17 | 11.1 | 2.2 | 4–14 | 104.96 | <.001 | .34 |
Note. M: mean; SD: standard deviation; R: range; for η2 a value of .10 is a small effect, .30 is a medium effect and .50 is a large effect.
Exploratory Analysis
To better understand the main effects of the Bike Smart program, follow-up exploratory analysis examined pre-test to post-test change at the individual item level. Logistic regression models with odds ratios (ORs) and 95% confidence intervals (CIs) were used to examine whether or not group membership could predict correct responses at the post-test after adjusting for the pre-test response rates. Of the 23 computerized items, 17 showed significantly greater gains for the treatment participants compared to the control participants (see Tables II and III). For example, the items testing the students’ ability to identify potentially dangerous cars in an intersection were highly significant, with the vast majority of treatment participants able to identify these critical threats at post-test. The significant differences were observed for both observational and computerized measures. For example, the treatment participants were 3.2 times more likely to place two fingers width from the eyebrows when securing their helmets. Likewise, the treatment participants were 6.9 times more likely to identify the first dangerous car in the computerized program compared to the control participants. For the observed helmet measures, the measure evaluating placement on the forehead two fingers above the eyebrows yielded a highly significant OR and the side to side placement measure was found to have an OR of 1.8, which approached statistical significance. Restriction of range was problematic for a few items. For example, the fastened helmet measure turned out to be scale-restricted had a ceiling effect with 90% of participants already fastening their helmet at pre-test. Consequently, this measure showed no change at post-test.
Table II.
Adjusted ORs for the Observational and Computerized Helmet and Safety Rules
Correct at Pre-test | Correct at Post-test | ||||||||||
---|---|---|---|---|---|---|---|---|---|---|---|
Treatment | Control | Treatment | Control | ||||||||
Outcome Measure | n | % | n | % | n | % | n | % | Adjusteda OR | p | 95% CI |
Observational bike helmet items | |||||||||||
Place two fingers from eyebrows | 52 | 48.6 | 37 | 37.4 | 65 | 60.7 | 32 | 32.3 | 3.2 | <.001 | 1.73–5.93 |
Sitting straight from ear to ear | 82 | 76.6 | 84 | 84.8 | 93 | 86.9 | 79 | 79.8 | 1.7 | .163 | 0.80–3.61 |
Buckle was strapped | 96 | 89.7 | 89 | 89.9 | 97 | 90.7 | 91 | 91.9 | 0.6 | .578 | 0.08–4.03 |
Computerized items | |||||||||||
Bike helmets | |||||||||||
Helmet too high | 18 | 16.7 | 19 | 18.8 | 103 | 95.4 | 22 | 21.8 | 159.5 | <.001 | 50.2–506.5 |
Helmet too low | 34.5 | 31.0 | 30 | 29.7 | 94 | 87.0 | 28 | 27.7 | 23.9 | <.001 | 10.8–52.8 |
Safety Rules | |||||||||||
Hand signal stop | 11 | 10.2 | 10 | 9.9 | 90 | 83.3 | 10 | 9.9 | 65.7 | <.001 | 25.1–172.1 |
Rider 1—no helmet | 87 | 80.6 | 80 | 79.2 | 99 | 91.7 | 82 | 81.2 | 2.8 | .027 | 1.1–6.8 |
Rider 2—safe | 101 | 93.5 | 96 | 95.0 | 103 | 95.4 | 94 | 93.1 | 1.5 | .469 | 0.5–5.1 |
Rider 3—stuff in hands | 92 | 85.2 | 83 | 82.2 | 106 | 98.1 | 86 | 85.1 | 10.6 | .003 | 2.2–50.9 |
Note. An OR of 1.5 is considered a small effect, 2.5 a medium effect, and 4.3 a large effect.
Table III.
Adjusted ORs for the Computerized Hazard Discrimination Items
Correct at Pre-test | Correct at Post-test | ||||||||||
---|---|---|---|---|---|---|---|---|---|---|---|
Treatment | Control | Treatment | Control | ||||||||
Outcome Measure | n | % | n | % | n | % | n | % | Adjusteda OR | p | 95% CI |
Dangerous car 1 | 42 | 36 | 93 | 51 | 3.4–14.0 | ||||||
Dangerous car 1 | 52 | 38.9 | 53 | 35.6 | 93 | 86.1 | 61 | 50.5 | 6.9 | <.001 | 8.4–102.0 |
Dangerous car 2 | 52 | 48.1 | 62 | 52.5 | 105 | 97.2 | 71 | 60.4 | 6.6 | <.001 | 2.5–17.3 |
Dangerous car 3 | 81 | 70.4 | 62 | 61.4 | 107 | 94.4 | 87 | 70.3 | 6.0 | .003 | 2.8–181.4 |
Sidewalk hazard—driveway | 68 | 30.6 | 66 | 34.7 | 84 | 99.1 | 32 | 86.1 | 22.6 | .003 | 4.9–18.7 |
Sidewalk hazard—pedestrian 2 | 33 | 30.6 | 88 | 34.7 | 84 | 77.8 | 96 | 31.7 | 9.5 | .275 | 0.5–10.8 |
Sidewalk hazard—car turn | 89 | 82.4 | 55 | 83.0 | 91 | 97.2 | 71 | 95.0 | 2.3 | .275 | 1.2–4.6 |
Sidewalk hazard—car turn | 62 | 63.9 | 67 | 54.5 | 97 | 84.3 | 76 | 70.3 | 2.3 | .019 | 1.4–7.7 |
Sidewalk hazard—car in street | 74 | 68.5 | 28 | 66.3 | 71 | 89.8 | 26 | 75.2 | 3.3 | .005 | 3.6–13.5 |
Sidewalk hazard—traffic light | 25 | 23.1 | 35 | 27.7 | 71 | 65.7 | 30 | 25.7 | 7.0 | <.001 | 3.6–16.5 |
Sidewalk hazard—pedestrian 3 | 34 | 31.5 | 91 | 34.7 | 69 | 63.9 | 94 | 29.7 | 7.7 | .556 | 0.4–5.1 |
Sidewalk hazard—pedestrian 3 | 95 | 88.9 | 92 | 90.1 | 102 | 94.4 | 94 | 93.1 | 1.5 | .556 | 1.3–56.3 |
Street hazard—car approaching 1 | 95 | 88.0 | 78 | 91.1 | 105 | 98.1 | 84 | 93.1 | 8.7 | .023 | 3.3–56.0 |
Street hazard—car approaching 1 | 82 | 75.9 | 71 | 77.2 | 90 | 97.2 | 80 | 83.2 | 13.0 | .538 | 0.6–2.6 |
Street hazard—car door | 80 | 74.1 | 78 | 70.3 | 97 | 83.3 | 86 | 79.2 | 1.3 | .538 | 0.7–4.2 |
Street hazard—pedestrian | 82 | 75.9 | 78 | 77.2 | 76 | 89.8 | 20 | 85.1 | 1.7 | .237 | 6.6–29.0 |
Street hazard—driveway | 19 | 17.6 | 25 | 24.8 | 76 | 70.4 | 58 | 19.8 | 1.4 | .209 | 0.8–2.6 |
Note. An OR of 1.5 is considered a small effect, 2.5 a medium effect, and 4.3 a large effect.
Discussion
The purpose of this evaluation was to determine if use of the Bike Smart program led to increased ability to identify and apply bicycling safety skills. In particular, we evaluated students’ ability to apply bike riding and related safety behaviors through both computerized and observational measures. The results of the evaluation demonstrated a significantly greater increase in the pretest to posttest scores for the treatment condition compared to the control condition representing a large overall effect size. The majority of individual computer-presented measures were significant. Regardless of gender, cohort, and grade the participants in the treatment condition showed greater gains than control participants in both the computer-presented items and the observational helmet measures. Overall, the results demonstrated that students could learn and apply bike safety information from the relatively brief yet targeted information contained in the the Bike Smart program. This revealed an advantage of the program over more traditional, lecture-based educational approaches which are time and staff intensive, and may not achieve measurable student outcomes. The Bike Smart program is designed to be completed within two class periods, and can be navigated relatively independently by most students. Furthermore, it offers the advantage of presenting a standard curriculum while providing individualized feedback to the student user. Thus, the Bike Smart program appears to be an effective and efficient approach to the educational component of bicycle safety.
In terms of identifying hazards to avoid while riding or crossing at an intersection, the results varied. On the items testing discrimination of dangerous cars in an intersection, the results were significant, providing replication of the effectiveness of these training items with a larger cohort of students than the previous evaluation with the companion program, “Walk Smart” (Glang et al., ). For identification of hazards while riding on the sidewalk, 78% (7/9) of the items were significant. However, for identification of hazards while on the street, only 40% (2/5) of the items were significant. This could potentially be attributed to greater saliency of some images than others. Overall, the results from these sections point to the need for additional instruction and training in real environments to teach students how to identify the hazards that appear suddenly while riding.
The result on the observational measure of helmet placement on the forehead was significant and points to transfer of knowledge gained in the computer environment to actual behavior. Incorrect helmet placement is a frequent error, with children often pushing the helmet up and off the forehead, thus exposing the frontal lobes of the brain to damage (Rivara et al., ). Students who viewed the program were more likely to correct this placement of the helmet following the training. In addition, the measure of helmet placement side to side approached significance. Interestingly, the measure of buckling the helmet was near ceiling at pre-test, suggesting that the importance of fastening a helmet is emphasized to children early on.
While overall the results of the program evaluation are highly promising, the study design included a number of limitations. First, this training program is designed to be one piece of a comprehensive package for bicycle safety including skills training, real-life training in outdoor environments, environmental controls, and adult supervision. In particular, the results of the measures for identifying hazards while riding were varied, pointing to the need for more training examples in real-life environments to assure mastery. Furthermore, children's lack of ability to manipulate the small and complex buckles found on helmets (determined through expert input and pilot testing) points to the need for adults to ensure adequate fit. Second, skill acquisition was evaluated through the computerized measures and behavioral observation of only the bike helmet placement, and project resources precluded conducting any follow-up assessment. In addition, the pre-test, post-test, and intervention were delivered in a brief time frame, with no follow up to evaluate knowledge retention. Additional research is necessary to determine whether project findings would maintain over time and to evaluate the effect of the program on student behavior while riding a bicycle, in order to demonstrate the transfer of safe riding behaviors and hazard discrimination training to real-life behavior. Furthermore, a longer period of time between the pre- and post treatment measures would limit the possibility of improvements related to practice effects. Third, although the average amount of time in each condition was approximately 20 min each day, data regarding exact exposure rates were not collected. Anecdotally, some students spent several minutes more than others using the Bike Smart program, due to slower response rates and/or higher error rates. Had this data been available, specific length of training might have been included as a covariate in the analysis. Fourth, data were not collected regarding the participants’ prior experience with helmets and riding bicycles. The results may not generalize to a broader sample of students if the evaluation sample had very limited degrees of familiarity with the program content. Fifth, the inter-observer agreement for the helmet measure was acceptable but not near perfect (as indicated by κ) due to variation on evaluating placement on the forehead. This points to the need for additional reliability training or an instrumental measure. Sixth, because students were randomly assigned to experimental condition, the statistical analyses were conducted at the student level and did not take into account the multi-level structure of the data (e.g., students nested within classrooms or schools). Finally, the strongest evidence for an injury prevention program lies in epidemiological data. This study was a randomized control trial; however, we used a geographically restricted sample (i.e., two schools in the Pacific Northwest) and measured skills knowledge and application. Further investigation with a larger, more diverse sample and evaluation of injury rates would provide greater evidence of the value of the program.
We believe the results suggest that the Bike Smart program can be an important component of safety training packages that include both skills-based and experiential training, and provide further support for the effectiveness of eHealth programs for the pediatric population. The main criticism of available behaviorally-based safety education programs involves their staff-intensive nature and related costs. The major advantage of an eHealth program to teach bicycle safety is the low cost and efficiency. As has been demonstrated in recent research with computer-generated environments such as virtual reality (Schwebel et al., ), if students can be taught key safety skills in a simulated environment, teachers can more efficiently translate these skills to examples in real environments. The obvious next step is to make available this type of safety education over the internet via eHealth sites, as has been accomplished in other pediatric health education efforts (e.g., Buller et al., ; Stinson et al., ). We believe this program is an improvement over other safety curricula given its success with teaching generalizable bicycle safety skills, and can form an important component of bicycle safety training in the schools.
Funding
Bike Safety Mcgruff
National Institute of Child Health and Human Development (grant R44 HD36554); National Institutes of Health.
Conflicts of Interest: None declared.
Acknowledgments
C.a.r.e.s Bike Safety Program Template
The authors would like to thank Carol Dennis, Damon Mitchell, Jeff Gau, Will Doolittle, Sherry Vondy Beaver, and Susan Schroeder for their assistance with program development and evaluation.
References
- Barton BK, Schwebel DC, Morrongiello BA. Brief report: Increasing safe pedestrian behaviors through simple skills training. Journal of Pediatric Psychology. 2007;32:475–480. [PubMed] [Google Scholar]
- Bike Smart. 2005. [Computer software]. Eugene, OR: Oregon Center for Applied Science. [Google Scholar]
- Buller DB, Borland R, Woodall WG, Hall JR, Hines JM, Burris-Woodall P, et al. Randomized trials on consider this, a tailored, internet-delivered smoking prevention program for adolescents. Health Education & Behavior. 2008;35:260–281.[PMC free article] [PubMed] [Google Scholar]
- Centers for Disease Control [CDC] WISQARS Leading Causes of Death Reports, 1999–2005. 2005a. Retrieved August 28, 2009, from http://webappa.cdc.gov/sasweb/ncipc/leadcaus10.html. [Google Scholar]
- Centers for Disease Control [CDC] WISQARS Injury Mortality Reports, 1999–2005. 2005b. Retrieved August 28, 2009, from http://webappa.cdc.gov/sasweb/ncipc/mortrate10_sy.html. [Google Scholar]
- Chen J, Kresnow M, Thomas S, Dellinger A. Injury-prevention counseling and behavior among US children: Results from the second injury control and risk survey. Pediatrics. 2007;119:958–965. [PubMed] [Google Scholar]
- Ching RP, Thompson DC, Thompson RS, Thomas DJ, Chilcott WC, Rivara FP. Damage to bicycle helmets involved with crashes. Annals of Accident Prevention. 1997;29:555–562. [PubMed] [Google Scholar]
- Cohen J. A coefficient of agreement for nominal scales. Educational and Psychological Measurement. 1960;20:37–46.[Google Scholar]
- DeBar L, Dickerson J, Clarke G, Stevens V, Ritenbaugh C, Aickin M. Using a website to build community and enhance outcomes in a group, multi-component intervention promoting healthy diet and exercise in adolescents. Journal of Pediatric Psychology. 2009;34:539–550.[PMC free article] [PubMed] [Google Scholar]
- Engelmann S, Carnine D. Theory of instruction: Principles and applications. NY: Irvington; 1982. [Google Scholar]
- Finnoff JT, Laskowski ER, Altman KL, Diehl NN. Barriers to bicycle helmet use. Pediatrics. 2001;108:e4. [PubMed] [Google Scholar]
- Finvers KA, Strother RT, Mohtadi N. The effect of bicycling helmets in preventing significant bicycle-related injuries in children. Clinical Journal of Sports Medicine. 1996;6:102–107. [PubMed] [Google Scholar]
- Gielen AC, Sleet D. Application of behavior-change theories and methods to brain injury prevention. Epidemiological Review. 2003;25:65–76. [PubMed] [Google Scholar]
- Glang A. Interactive program to teach bicycle safety. 1999. Grant from National Institute of Child Health and Human Development, R43 HD36554-01. [Google Scholar]
- Glang A, Noell J, Ary D, Swartz L. Using interactive multimedia to teach pedestrian safety: An exploratory study. American Journal of Health Behavior. 2005;29:435–442. [PubMed] [Google Scholar]
- Haileyesus T, Annest JL, Dellinger AM. Cyclists injured while sharing the road with motor vehicles. Injury Prevention. 2007;13:202–206.[PMC free article] [PubMed] [Google Scholar]
- Horner RH, McDonnell JJ, Bellamy GT. Teaching generalized skills: General case instruction in simulation and community settings. In: Horner RH, Meyer LH, Fredericks HDB, editors. Education of learners with severe handicaps: Exemplary service strategies. Baltimore, MD: Paul H. Brookes Publishing; 1986. pp. 289–310. [Google Scholar]
- Hornung RL, Lennon PA, Garrett JM, DeVellis RF, Weinberg PD, Strecher VJ. Interactive computer technology for skin cancer prevention targeting children. American Journal of Preventive Medicine. 2000;18:69–76. [PubMed] [Google Scholar]
- Hotz G, Cohn S, Castelblanco A, Colston S, Thomas M, Weiss A, Nelson J, Duncan R. WalkSafe: A school-based pedestrian safety intervention program. Traffic Injury Prevention. 2004;5:382–389. [PubMed] [Google Scholar]
- Kirsch SE, Pullen N. Evaluation of a school-based education program to promote bicycle safety. Health Promotion Practice. 2003;4:138–145. [PubMed] [Google Scholar]
- Krishna S, Francisco BD, Balas EA, Konig P, Graff GR, Madsen RW. Internet-enabled interactive multimedia asthma education program: A randomized trial. Pediatrics. 2003;111:503–510. [PubMed] [Google Scholar]
- Landis JR, Koch GG. The measurement of observer agreement for categorical data. Biometrics. 1977;33:159–174. [PubMed] [Google Scholar]
- Luria JW, Smith GA, Chapman JI. An evaluation of a safety education program for kindergarten and elementary school children. Archives Of Pediatrics & Adolescent Medicine. 2000;154:227–231. [PubMed] [Google Scholar]
- Mish M, Barker M, (Directors/Producers) Be cool, play it safe (Motion Picture) Eugene, OR: EMP International Productions; 2000. [Google Scholar]
- Morris BA, Trimble NE, Fendley SJ. Increasing bicycle helmet use in the community: Measuring response to a wide-scale, 2-year effort. Canadian Family Physician. 1994;40:1126–1131.[PMC free article] [PubMed] [Google Scholar]
- Morrongiello BA. Unintentional injury prevention: Priorities for research, capacity building, and knowledge transfer. Guelph ON: Canadian Institutes of Health Research and the Canadian Injury Research network; 2003. [Google Scholar]
- Morrongiello BA, Kiriakou S. Evaluation of the effectiveness of single-session school-based programmes to increase childrens’ seat belt and pedestrian safety knowledge and self-reported behaviours. International Journal of Injury Control and Safety Promotion. 2006;13:15–25. [PubMed] [Google Scholar]
- Morrongiello BA, Schwebel DC. Gaps in childhood injury research and prevention: What can developmental scientists contribute? Child Development Perspectives. 2008;2:78–84.[Google Scholar]
- Nagel RW, Hankenhof BJ, Kimmel S, Saxe J. Educating grade school children using a structured bicycle safety program. Journal of Trauma: Injury, Infection, and Critical Care. 2003;55:920–923. [PubMed] [Google Scholar]
- National Highway Traffic Safety Administration (NHTSA) Traffic safety facts: 2006 data. Washington, DC: NHTSA National Center for Statistics and Analysis; 2006. [Google Scholar]
- National SAFE KIDS Campaign (NSKC) Bicycle injury fact sheet. Washington, DC: NSKC; 2004. [Google Scholar]
- Peterson L, Schick B. Empirically derived injury prevention rules. Journal of Applied Behavior Analysis. 1993;26:451–460.[PMC free article] [PubMed] [Google Scholar]
- Rivara FP, Astley SJ, Clarren SK, Thompson DC, Thompson RS. Fit of bicycle safety helmets and risk of head injuries in children. Injury Prevention. 1999;5:194–197.[PMC free article] [PubMed] [Google Scholar]
- Rivara FP, Thompson DC, Thompson RS. Epidemiology of bicycle injuries and risk factors for serious injury. Injury Prevention. 1997;3:110–114.[PMC free article] [PubMed] [Google Scholar]
- Rivara FP, Thompson DC, Thompson RS, Rogers LW, Alexander B, Felix D, Bergman AB. The Seattle children's bicycle helmet campaign: Changes in helmet use and head injury admissions. Pediatrics. 1994;93:567–569. [PubMed] [Google Scholar]
- Royal S, Kendrick D, Coleman T. Promoting bicycle helmet wearing by children using non-legislative interventions: Systematic review and meta-analysis. Injury Prevention. 2007;13:162–167.[PMC free article] [PubMed] [Google Scholar]
- Schwebel DC, Gaines J, Severson J. Validation of virtual reality as a tool to understand and prevent child pedestrian injury. Annals of Accident Prevention. 2008;40:1394–1400. [PubMed] [Google Scholar]
- Stinson J, Wilson R, Gill N, Yamada J, Holt J. A systematic review of internet-based self-management interventions for youth with health conditions. Journal of Pediatric Psychology. 2009;34:495–510. [PubMed] [Google Scholar]
- Thompson DC, Rivara FP, Thompson RS. Effectiveness of bicycle safety helmets in preventing head injuries. Journal of American Medical Association. 1996;276:1968–1973. [PubMed] [Google Scholar]
- Van Houten R, Van Houten J, Malenfant JE. Impact of a comprehensive safety program on bicycle helmet use among middle-school children. Journal of Applied Behavior Analysis. 2007;40:239–247.[PMC free article] [PubMed] [Google Scholar]
- Walters ST, Wright JA, Shegog R. A review of computer and Internet-based interventions for smoking behavior. Addictive Behaviors. 2006;31:264–277. [PubMed] [Google Scholar]
- Wang Y, Nihan NL. Estimating the risk of collisions between bicycles and motor vehicles at signalized intersections. Accident Analysis and Prevention. 2004;36:313–327. [PubMed] [Google Scholar]